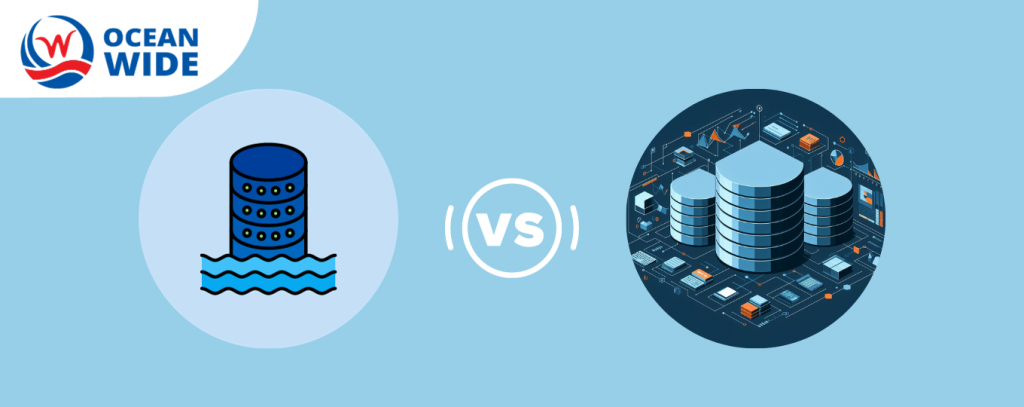
Embark on a journey with Ocean Wide to uncover the essence of “What is Data Lake?“. Discover the pivotal role of data lakes and their distinctions from Data Warehouses. Explore the significance and nuances of these large data storage solutions, unraveling why Data Lake matters in today’s dynamic data landscape.
What is a data lake?
A data lake is a centralized repository designed to store, process, and secure large amounts of structured, semi-structured, and unstructured data. It can store data in its native format and process any variety of it, without size limits.
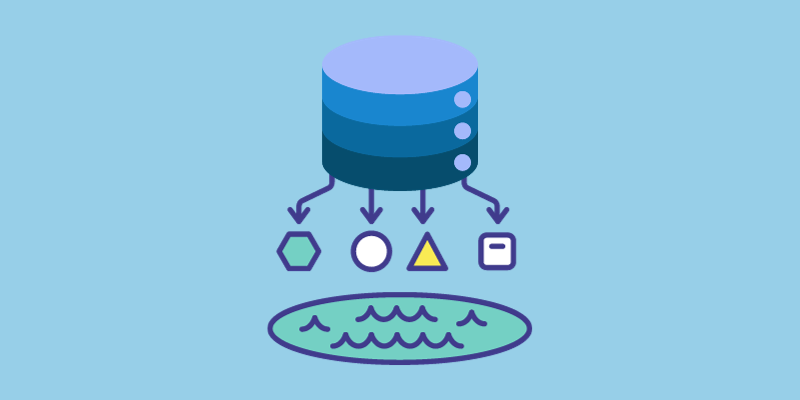
This platform provides scalability and security, enabling enterprises to ingest data from any system at any speed, whether it’s on-premises, cloud, or edge-computing systems. It can store any type or volume of data in full fidelity, process data in real time or batch mode, and analyze data using SQL, Python, R, or any other language, third-party data, or analytics application.
It can store structured, unstructured, and semi-structured data at any scale, and also store data in its original format without any strict requirements, with no limit on capacity, records, or number of files. This allows users to use different data formats while increasing their ability to analyze on different platforms. Nowadays, it is widely applied in the field of data science, which requires huge amounts of data and modern analytical techniques such as predictive models, data mining, and machine learning.
The benefits of Data Lake
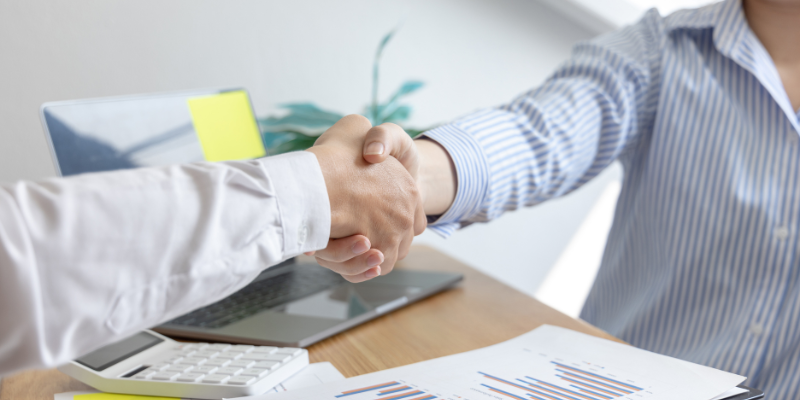
The outstanding advantage of Data Lake is its ability to exploit many types of data from different sources in a short time while giving users the right to collaborate and analyze data in different ways, helping to make decisions quickly and accurately.
Here are some key benefits:
Enhancing customer interaction: It can merge customer data from CRMs with media and e-commerce platforms, including purchase history and trouble tickets. This enables businesses to identify the most profitable customer groups, understand the causes of customer abandonment, and offer incentives that can help increase customer loyalty.
Boosting R&D innovation choices: It aids R&D teams in testing their hypotheses, adjusting their assumptions, and evaluating results to accelerate work efficiency.
Increasing operational efficiency: The Internet of Things (IoT) offers numerous methods to collect data on production processes through real-time data from Internet-connected devices. It simplifies the storage and analytics of IoT data and helps discover new methods to reduce operating costs and increase quality.
The applications of Data Lake
Data management and data governance
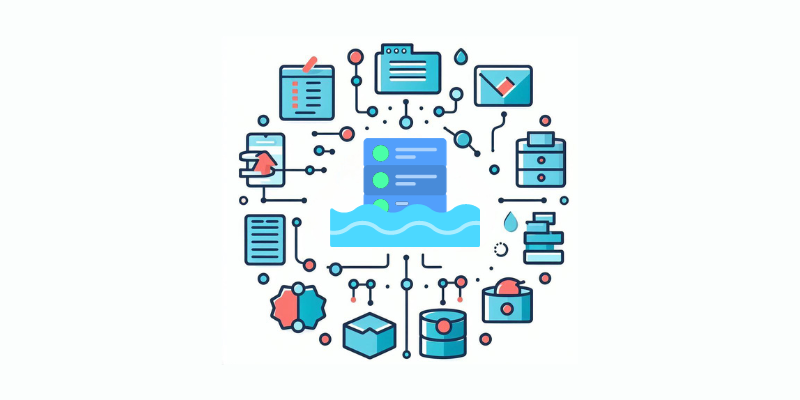
As we have learned above, Data Lake is a place that contains many types of data, including sensitive data or data that needs to comply with certain requirements, which can make users worry about security issues. Because there are no tables like a database, the permissions are flexible but difficult to set up, and these permissions must be based on specific objects or metadata definitions.
Nowadays, this problem can be solved by various management tools, helping businesses control who has access to the data. Data catalog solutions allow creating data catalogs, specifying different types of data, and controlling access and storage policies for each type.
Storing multiple copies of data
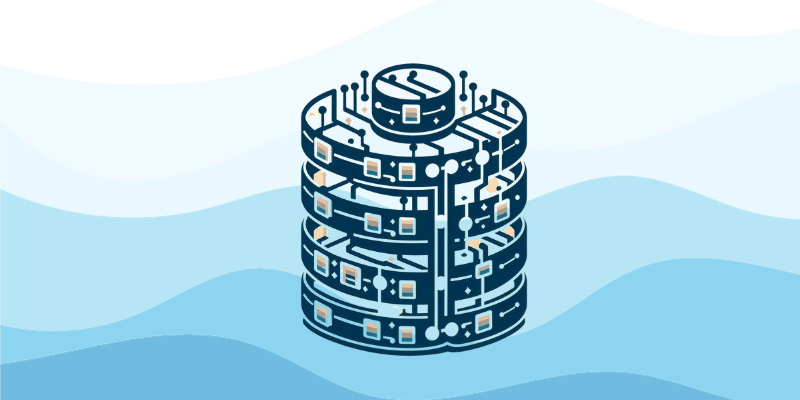
Data Lake allows storing unstructured data and many other types of data separately from computers, allowing users to store large amounts of data with low investment costs. Typically, data lakes are used to store both raw and processed data. The needs for processing raw data may include: System validation, data flow Error recovery Exploratory analysis In addition, there is also processed data that is used in the analysis process. This data also needs to be stored to serve the purpose of analysis in the future, as well as the basis for reports and dashboards.
Nowadays, data lakes can solve problems that databases could not solve in the past. Accordingly, storing data in databases is quite cumbersome and expensive, so storing both historical and current data is almost impossible. Data lakes today have high scalability and can store almost unlimitedly at low cost. Not only that, but Data Lake also allows users to store multiple copies of data for different purposes.
Implementing storage policies
Data lakes usually store historical data but cannot store all data forever. Data that is no longer needed will be processed according to standards such as EU GDPR, California CCPA, etc. to save maximum memory. In addition, there needs to be a technical method to separate the data to be deleted from the data to be retained. Otherwise, locating data on the Data Lake storage architecture (which may include storage services such as Amazon S3, HDFS, and block storage devices) will be quite complicated. The problem can be solved by data catalog solutions, which provide a central interface to classify data according to the desired storage time.
Data Lake architecture
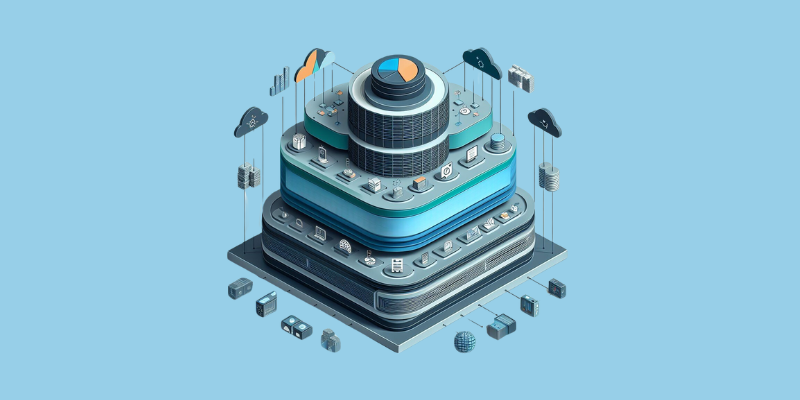
Data Lake architecture can be divided into six parts:
Ingestion Tier: The tiers on the left describe the data sources. Data can be loaded into Data Lake in batches or in real time.
Insights Tier: The tiers on the right represent the research side, where detailed information from the system is used. SQL, NoSQL, or even Excel queries can be used to analyze data.
HDFS is a cost-effective solution for both structured and unstructured data. It is the “landing zone” for all data in the system.
The distillation tier takes data from the storage tire and converts it into structured data for easier analysis.
The processing tier runs analytical algorithms and user queries with different real-time, interactive, and batch modes to create structured data for easier analysis.
The unified operations tier manages and monitors the system. It includes auditing and master management, data management, and workflow management.
Differentiating Data Lake and Data Warehouse
Data Lake and Data Warehouse are both used to store large data and are often confused. Data Lake is a large, raw Data Warehouse, whose purpose is still undefined. Data Warehouse is a structured data storage, filtered, processed for a specific purpose. There is even a new trend of Data Lake house data management architecture, combining the flexibility of Data Lake with the data management capabilities of Data Warehouse.
The key differences between a data lake and a data warehouse are as follows:
Parameters | Data Lake | Data Warehouse |
Data | Store everything | Focus only on Business Processes |
Processing | Data is mostly unprocessed | Data is highly processed |
Data type | Raw (all types, no matter source of structure) | Processed (data stored according to metrics and attributes) |
Task | Share data stewardship | Optimized for data retrieval |
Agility | Very agile, configure and reconfigure if needed | Compared to Data Lake, it is less flexible and has a fixed configuration |
Users | Data scientists, those who need in-depth analysis and tools (such as predictive modeling) to understand it | Business professionals, those who need it for operations |
Warehouse | Designed Data Lake to store at low cost | Expensive memory with fast response time is used |
Security | Provides lower control capability | Allows better data control |
Replace EDW | Data Lake can be a source for EDW | Supplement for EDW (not replace) |
Schema | Schema on read (no predefined schema) | Schema on write (predefined schema) |
Accessibility | Accessible and easy to update | Complicated to make changes |
Level of detail of the data | Data at the level of detail or low detail | Data at the summary or aggregate level of detail |
Tools | Can use open source / tools like Hadoop / Map Reduce | Mainly commercial tools |
Conclusion
Data Lake and Data Warehouse are solutions for individuals and organizations that can store and work with large amounts of data. However, it is important to identify which data suits us best based on the types of data we work with, what we want to do with the data, the complexity of the data collection process, and our strategy to manage and govern the data, as well as the tools and skills available in our organization. Through this article, Ocean Wide hopes that you can understand the difference between these two solutions and find the best one for yourself.